Mostrar el registro sencillo del ítem
Improved personalized survival prediction of patients with diffuse large B-cell Lymphoma using gene expression profiling
dc.contributor.author | MOSQUERA ORGUEIRA, ADRIAN | |
dc.contributor.author | Díaz Arias, José | |
dc.contributor.author | Cid López, Miguel | |
dc.contributor.author | Peleteiro Raindo, Andrés | |
dc.contributor.author | Antelo Rodríguez, Beatriz | |
dc.contributor.author | Aliste Santos, Carlos | |
dc.contributor.author | Alonso Vence, Natalia | |
dc.contributor.author | Bendaña López, Mª Ángeles | |
dc.contributor.author | Abuin Blanco, Aitor | |
dc.contributor.author | BAO PEREZ, LAURA | |
dc.contributor.author | González Pérez, Marta Sonia | |
dc.contributor.author | Pérez Encinas, Manuel Mateo | |
dc.contributor.author | Fraga Rodríguez, Máximo Francisco | |
dc.contributor.author | Bello López, José Luis | |
dc.date.accessioned | 2022-04-26T07:43:56Z | |
dc.date.available | 2022-04-26T07:43:56Z | |
dc.date.issued | 2020 | |
dc.identifier.issn | 1471-2407 | |
dc.identifier.other | https://www.ncbi.nlm.nih.gov/pubmed/33087075 | es |
dc.identifier.uri | http://hdl.handle.net/20.500.11940/16539 | |
dc.description.abstract | BACKGROUND: Thirty to forty percent of patients with Diffuse Large B-cell Lymphoma (DLBCL) have an adverse clinical evolution. The increased understanding of DLBCL biology has shed light on the clinical evolution of this pathology, leading to the discovery of prognostic factors based on gene expression data, genomic rearrangements and mutational subgroups. Nevertheless, additional efforts are needed in order to enable survival predictions at the patient level. In this study we investigated new machine learning-based models of survival using transcriptomic and clinical data. METHODS: Gene expression profiling (GEP) of in 2 different publicly available retrospective DLBCL cohorts were analyzed. Cox regression and unsupervised clustering were performed in order to identify probes associated with overall survival on the largest cohort. Random forests were created to model survival using combinations of GEP data, COO classification and clinical information. Cross-validation was used to compare model results in the training set, and Harrel's concordance index (c-index) was used to assess model's predictability. Results were validated in an independent test set. RESULTS: Two hundred thirty-three and sixty-four patients were included in the training and test set, respectively. Initially we derived and validated a 4-gene expression clusterization that was independently associated with lower survival in 20% of patients. This pattern included the following genes: TNFRSF9, BIRC3, BCL2L1 and G3BP2. Thereafter, we applied machine-learning models to predict survival. A set of 102 genes was highly predictive of disease outcome, outperforming available clinical information and COO classification. The final best model integrated clinical information, COO classification, 4-gene-based clusterization and the expression levels of 50 individual genes (training set c-index, 0.8404, test set c-index, 0.7942). CONCLUSION: Our results indicate that DLBCL survival models based on the application of machine learning algorithms to gene expression and clinical data can largely outperform other important prognostic variables such as disease stage and COO. Head-to-head comparisons with other risk stratification models are needed to compare its usefulness. | en |
dc.rights | Atribución 4.0 Internacional | |
dc.rights.uri | http://creativecommons.org/licenses/by/4.0/ | |
dc.subject.mesh | Survival Analysis | * |
dc.subject.mesh | Middle Aged | * |
dc.subject.mesh | RNA-Binding Proteins | * |
dc.subject.mesh | Survival | * |
dc.subject.mesh | Gene Expression Profiling | * |
dc.subject.mesh | Microarray Analysis | * |
dc.subject.mesh | Computational Biology | * |
dc.subject.mesh | Humans | * |
dc.subject.mesh | Lymphoma | * |
dc.subject.mesh | Gene Expression Regulation | * |
dc.subject.mesh | Retrospective Studies | * |
dc.subject.mesh | bcl-X Protein | * |
dc.subject.mesh | Prognosis | * |
dc.title | Improved personalized survival prediction of patients with diffuse large B-cell Lymphoma using gene expression profiling | en |
dc.type | Journal Article | es |
dc.authorsophos | Mosquera Orgueira, A.;Díaz Arias, J.;Cid López, M.;Peleteiro Raíndo, A.;Antelo Rodríguez, B.;Aliste Santos, C.;Alonso Vence, N.;Bendaña López, Á;Abuín Blanco, A.;Bao Pérez, L.;González Pérez, M. S.;Pérez Encinas, M. M.;Fraga Rodríguez, M. F.;Bello López, J. L. | |
dc.identifier.doi | 10.1186/s12885-020-07492-y | |
dc.identifier.pmid | 33087075 | |
dc.identifier.sophos | 39251 | |
dc.issue.number | 1 | es |
dc.journal.title | BMC CANCER | es |
dc.organization | Servizo Galego de Saúde::Estrutura de Xestión Integrada (EOXI)::EOXI de Santiago de Compostela - Complexo Hospitalario Universitario de Santiago de Compostela::Anatomía Patolóxica | es |
dc.organization | Servizo Galego de Saúde::Estrutura de Xestión Integrada (EOXI)::EOXI de Santiago de Compostela - Complexo Hospitalario Universitario de Santiago de Compostela::Hematoloxía clínica||SERGAS::Área Sanitaria de Santiago de Compostela e Barbanza::IDIS.- Instituto de investigaciones sanitarias de Santiago | es |
dc.organization | Servizo Galego de Saúde::Estrutura de Xestión Integrada (EOXI)::Instituto de Investigación Sanitaria de Santiago de Compostela (IDIS) | es |
dc.page.initial | 1017 | es |
dc.rights.accessRights | openAccess | |
dc.subject.decs | pronóstico | * |
dc.subject.decs | linfoma | * |
dc.subject.decs | análisis por micromatrices | * |
dc.subject.decs | estudios retrospectivos | * |
dc.subject.decs | mediana edad | * |
dc.subject.decs | análisis de supervivencia | * |
dc.subject.decs | regulación de la expresión génica | * |
dc.subject.decs | supervivencia | * |
dc.subject.decs | biología computacional | * |
dc.subject.decs | proteínas de unión al ARN | * |
dc.subject.decs | humanos | * |
dc.subject.decs | perfiles de expresión génica | * |
dc.subject.decs | proteína bcl-X | * |
dc.subject.keyword | CHUS | es |
dc.subject.keyword | IDIS | es |
dc.typefides | Artículo Original | es |
dc.typesophos | Artículo Original | es |
dc.volume.number | 20 | es |
Ficheros en el ítem
Este ítem aparece en la(s) siguiente(s) colección(ones)
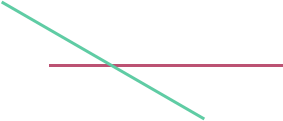