Mostrar o rexistro simple do ítem
Machine learning classification models for fetal skeletal development performance prediction using maternal bone metabolic proteins in goats
dc.contributor.author | Liu, Yong | |
dc.contributor.author | MUNTEANU -, CRISTIAN ROBERT | |
dc.contributor.author | Yan, Qiongxian | |
dc.contributor.author | Pedreira, Nieves | |
dc.contributor.author | Kang, Jinhe | |
dc.contributor.author | Tang, Shaoxun | |
dc.contributor.author | Zhou, Chuanshe | |
dc.contributor.author | He, Zhixiong | |
dc.contributor.author | Tan, Zhiliang | |
dc.date.accessioned | 2022-01-25T12:15:53Z | |
dc.date.available | 2022-01-25T12:15:53Z | |
dc.date.issued | 2019 | |
dc.identifier.issn | 2167-8359 | |
dc.identifier.other | https://www.ncbi.nlm.nih.gov/pubmed/31649832 | es |
dc.identifier.other | https://www.ncbi.nlm.nih.gov/pmc/articles/PMC6802673/pdf/peerj-07-7840.pdf | es |
dc.identifier.uri | http://hdl.handle.net/20.500.11940/15902 | |
dc.description.abstract | Background: In developing countries, maternal undernutrition is the major intrauterine environmental factor contributing to fetal development and adverse pregnancy outcomes. Maternal nutrition restriction (MNR) in gestation has proven to impact overall growth, bone development, and proliferation and metabolism of mesenchymal stem cells in offspring. However, the efficient method for elucidation of fetal bone development performance through maternal bone metabolic biochemical markers remains elusive. Methods: We adapted goats to elucidate fetal bone development state with maternal serum bone metabolic proteins under malnutrition conditions in mid- and late-gestation stages. We used the experimental data to create 72 datasets by mixing different input features such as one-hot encoding of experimental conditions, metabolic original data, experimental-centered features and experimental condition probabilities. Seven Machine Learning methods have been used to predict six fetal bone parameters (weight, length, and diameter of femur/humerus). Results: The results indicated that MNR influences fetal bone development (femur and humerus) and fetal bone metabolic protein levels (C-terminal telopeptides of collagen I, CTx, in middle-gestation and N-terminal telopeptides of collagen I, NTx, in late-gestation), and maternal bone metabolites (low bone alkaline phosphatase, BALP, in middle-gestation and high BALP in late-gestation). The results show the importance of experimental conditions (ECs) encoding by mixing the information with the serum metabolic data. The best classification models obtained for femur weight (Fw) and length (FI), and humerus weight (Hw) are Support Vector Machines classifiers with the leave-one-out cross-validation accuracy of 1. The rest of the accuracies are 0.98, 0.946 and 0.696 for the diameter of femur (Fd), diameter and length of humerus (Hd, Hl), respectively. With the feature importance analysis, the moving averages mixed ECs are generally more important for the majority of the models. The moving average of parathyroid hormone (PTH) within nutritional conditions (MA-PTH-experim) is important for Fd, Hd and Hl prediction models but its removal for enhancing the Fw, Fl and Hw model performance. Further, using one feature models, it is possible to obtain even more accurate models compared with the feature importance analysis models. In conclusion, the machine learning is an efficient method to confirm the important role of PTH and BALP mixed with nutritional conditions for fetal bone growth performance of goats. All the Python scripts including results and comments are available into an open repository at https://gitlab.com/muntisa/goat-bones-machine-learning. | en |
dc.language.iso | eng | es |
dc.rights | Atribución 4.0 Internacional | * |
dc.rights.uri | http://creativecommons.org/licenses/by/4.0/ | * |
dc.title | Machine learning classification models for fetal skeletal development performance prediction using maternal bone metabolic proteins in goats | en |
dc.type | Artigo | es |
dc.identifier.doi | 10.7717/peerj.7840 | |
dc.identifier.pmid | 31649832 | |
dc.identifier.sophos | 32285 | |
dc.journal.title | PeerJ | es |
dc.organization | Servizo Galego de Saúde::Estrutura de Xestión Integrada (EOXI)::Instituto de Investigación Biomédica da Coruña (INIBIC) | es |
dc.rights.accessRights | openAccess | es |
dc.subject.keyword | INIBIC | es |
dc.typefides | Artículo Original | es |
dc.typesophos | Artículo Original | es |
dc.volume.number | 7 | es |
Ficheiros no ítem
Este ítem aparece na(s) seguinte(s) colección(s)
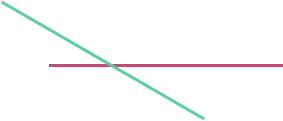