Mostrar el registro sencillo del ítem
Improvement of Epitope Prediction Using Peptide Sequence Descriptors and Machine Learning
dc.contributor.author | MUNTEANU -, CRISTIAN ROBERT | |
dc.contributor.author | Gestal, Marcos | |
dc.contributor.author | Martinez-Acevedo, Yunuen G | |
dc.contributor.author | Pedreira, Nieves | |
dc.contributor.author | PAZOS SIERRA, ALEJANDRO | |
dc.contributor.author | Dorado, Julian | |
dc.date.accessioned | 2022-01-25T12:16:19Z | |
dc.date.available | 2022-01-25T12:16:19Z | |
dc.date.issued | 2019 | |
dc.identifier.issn | 1661-6596 | |
dc.identifier.other | https://www.ncbi.nlm.nih.gov/pubmed/31491969 | es |
dc.identifier.uri | http://hdl.handle.net/20.500.11940/15907 | |
dc.description.abstract | In this work, we improved a previous model used for the prediction of proteomes as new B-cell epitopes in vaccine design. The predicted epitope activity of a queried peptide is based on its sequence, a known reference epitope sequence under specific experimental conditions. The peptide sequences were transformed into molecular descriptors of sequence recurrence networks and were mixed under experimental conditions. The new models were generated using 709,100 instances of pair descriptors for query and reference peptide sequences. Using perturbations of the initial descriptors under sequence or assay conditions, 10 transformed features were used as inputs for seven Machine Learning methods. The best model was obtained with random forest classifiers with an Area Under the Receiver Operating Characteristics (AUROC) of 0.981 +/- 0.0005 for the external validation series (five-fold cross-validation). The database included information about 83,683 peptides sequences, 1448 epitope organisms, 323 host organisms, 15 types of in vivo processes, 28 experimental techniques, and 505 adjuvant additives. The current model could improve the in silico predictions of epitopes for vaccine design. The script and results are available as a free repository. | en |
dc.language.iso | eng | es |
dc.rights | Atribución 4.0 Internacional | * |
dc.rights.uri | http://creativecommons.org/licenses/by/4.0/ | * |
dc.subject.mesh | Humans | * |
dc.subject.mesh | Amino Acid Sequence | * |
dc.subject.mesh | Peptides | * |
dc.subject.mesh | Epitope Mapping | * |
dc.subject.mesh | ROC Curve | * |
dc.subject.mesh | Structure-Activity Relationship | * |
dc.title | Improvement of Epitope Prediction Using Peptide Sequence Descriptors and Machine Learning | en |
dc.type | Artigo | es |
dc.identifier.doi | 10.3390/ijms20184362 | |
dc.identifier.pmid | 31491969 | |
dc.identifier.sophos | 32302 | |
dc.issue.number | 18 | es |
dc.journal.title | INTERNATIONAL JOURNAL OF MOLECULAR SCIENCES | es |
dc.organization | Servizo Galego de Saúde::Estrutura de Xestión Integrada (EOXI)::Instituto de Investigación Biomédica da Coruña (INIBIC) | es |
dc.relation.publisherversion | https://res.mdpi.com/d_attachment/ijms/ijms-20-04362/article_deploy/ijms-20-04362.pdf | es |
dc.rights.accessRights | openAccess | es |
dc.subject.decs | curva ROC | * |
dc.subject.decs | mapeo de epítopos | * |
dc.subject.decs | péptidos | * |
dc.subject.decs | humanos | * |
dc.subject.decs | relación estructura-actividad | * |
dc.subject.decs | secuencia de aminoácidos | * |
dc.subject.keyword | INIBIC | es |
dc.typefides | Artículo Original | es |
dc.typesophos | Artículo Original | es |
dc.volume.number | 20 | es |
Ficheros en el ítem
Este ítem aparece en la(s) siguiente(s) colección(ones)
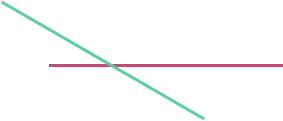