Mostrar el registro sencillo del ítem
Deep Convolutional Approaches for the Analysis of COVID-19 Using Chest X-Ray Images From Portable Devices
dc.contributor.author | de Moura Ramos, Jose Joaquim | |
dc.contributor.author | Ramos García, Lucía | |
dc.contributor.author | Lizancos Vidal, Plácido Francisco | |
dc.contributor.author | Cruz Sánchez, Nilfa Milena | |
dc.contributor.author | Abelairas López, Lucia | |
dc.contributor.author | Castro López, Eva | |
dc.contributor.author | Novo Buján, Jorge | |
dc.contributor.author | Ortega Hortas, Marcos | |
dc.date.accessioned | 2022-04-12T11:37:17Z | |
dc.date.available | 2022-04-12T11:37:17Z | |
dc.date.issued | 2020 | |
dc.identifier.issn | 2169-3536 | |
dc.identifier.other | https://www.ncbi.nlm.nih.gov/pubmed/34786295 | es |
dc.identifier.uri | http://hdl.handle.net/20.500.11940/16453 | |
dc.description.abstract | The recent human coronavirus disease (COVID-19) is a respiratory infection caused by severe acute respiratory syndrome coronavirus 2 (SARS-CoV-2). Given the effects of COVID-19 in pulmonary tissues, chest radiography imaging plays an important role in the screening, early detection, and monitoring of the suspected individuals. Hence, as the pandemic of COVID-19 progresses, there will be a greater reliance on the use of portable equipment for the acquisition of chest X-ray images due to its accessibility, widespread availability, and benefits regarding to infection control issues, minimizing the risk of cross-contamination. This work presents novel fully automatic approaches specifically tailored for the classification of chest X-ray images acquired by portable equipment into 3 different clinical categories: normal, pathological, and COVID-19. For this purpose, 3 complementary deep learning approaches based on a densely convolutional network architecture are herein presented. The joint response of all the approaches allows to enhance the differentiation between patients infected with COVID-19, patients with other diseases that manifest characteristics similar to COVID-19 and normal cases. The proposed approaches were validated over a dataset specifically retrieved for this research. Despite the poor quality of the chest X-ray images that is inherent to the nature of the portable equipment, the proposed approaches provided global accuracy values of 79.62%, 90.27% and 79.86%, respectively, allowing a reliable analysis of portable radiographs to facilitate the clinical decision-making process. | en |
dc.rights | Attribution-NonCommercial-NoDerivatives 4.0 International | |
dc.rights.uri | https://creativecommons.org/licenses/by-nc-nd/4.0/ | |
dc.title | Deep Convolutional Approaches for the Analysis of COVID-19 Using Chest X-Ray Images From Portable Devices | en |
dc.type | Journal Article | es |
dc.authorsophos | De Moura, J Garcia, LR Vidal, PFL Cruz, M Lopez, LA Lopez, EC Novo, J Ortega, M | |
dc.identifier.doi | 10.1109/ACCESS.2020.3033762 | |
dc.identifier.pmid | PMC8545263 | |
dc.identifier.sophos | 38845 | |
dc.journal.title | IEEE access : practical innovations, open solutions. | es |
dc.organization | Servizo Galego de Saúde::Estrutura de Xestión Integrada (EOXI)::EOXI de A Coruña - Complexo Hospitalario Universitario de A Coruña::Radiodiagnóstico | es |
dc.organization | Servizo Galego de Saúde::Estrutura de Xestión Integrada (EOXI)::Instituto de Investigación Biomédica da Coruña (INIBIC) | es |
dc.page.initial | 195594 | es |
dc.page.final | 195607 | es |
dc.rights.accessRights | openAccess | |
dc.subject.keyword | CHUAC | es |
dc.subject.keyword | INIBIC | es |
dc.typefides | Artículo Original | es |
dc.typesophos | Artículo Original | es |
dc.volume.number | 8. | es |
Ficheros en el ítem
Este ítem aparece en la(s) siguiente(s) colección(ones)
Excepto si se señala otra cosa, la licencia del ítem se describe como Attribution-NonCommercial-NoDerivatives 4.0 International
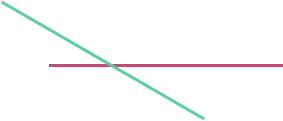