A neural network for prediction of risk of nosocomial infection at intensive care units: a didactic preliminary model
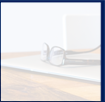
Identificadores
Identificadores
Visualización o descarga de ficheros
Visualización o descarga de ficheros
Fecha de publicación
2020Título de revista
EINSTEIN (SAO PAULO)
Tipo de contenido
Journal Article
DeCS
algoritmos | evaluación de riesgos | humanos | unidades de cuidados intensivos | índice APACHEMeSH
Risk Assessment | Humans | Intensive Care Units | APACHE | AlgorithmsResumen
OBJECTIVE: To propose a preliminary artificial intelligence model, based on artificial neural networks, for predicting the risk of nosocomial infection at intensive care units. METHODS: An artificial neural network is designed that employs supervised learning. The generation of the datasets was based on data derived from the Japanese Nosocomial Infection Surveillance system. It is studied how the Java Neural Network Simulator learns to categorize these patients to predict their risk of nosocomial infection. The simulations are performed with several backpropagation learning algorithms and with several groups of parameters, comparing their results through the sum of the squared errors and mean errors per pattern. RESULTS: The backpropagation with momentum algorithm showed better performance than the backpropagation algorithm. The performance improved with the xor. README file parameter values compared to the default parameters. There were no failures in the categorization of the patients into their risk of nosocomial infection. CONCLUSION: While this model is still based on a synthetic dataset, the excellent performance observed with a small number of patterns suggests that using higher numbers of variables and network layers to analyze larger volumes of data can create powerful artificial neural networks, potentially capable of precisely anticipating nosocomial infection at intensive care units. Using a real database during the simulations has the potential to realize the predictive ability of this model.
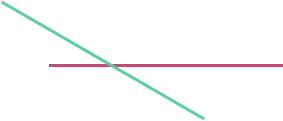