Mostrar el registro sencillo del ítem
CT Radiomics in Colorectal Cancer: Detection of KRAS Mutation Using Texture Analysis and Machine Learning
dc.contributor.author | Gonzalez-Castro, V. | |
dc.contributor.author | Cernadas, E. | |
dc.contributor.author | HUELGA ZAPICO, EMILIO | |
dc.contributor.author | Fernandez-Delgado, M. | |
dc.contributor.author | Porto, J. | |
dc.contributor.author | Antúnez López, José Ramón | |
dc.contributor.author | Souto Bayarri, José Miguel | |
dc.date.accessioned | 2022-04-29T10:28:12Z | |
dc.date.available | 2022-04-29T10:28:12Z | |
dc.date.issued | 2020 | |
dc.identifier.issn | 2076-3417 | |
dc.identifier.uri | http://hdl.handle.net/20.500.11940/16635 | |
dc.description.abstract | In this work, by using descriptive techniques, the characteristics of the texture of the CT (computed tomography) image of patients with colorectal cancer were extracted and, subsequently, classified in KRAS+ or KRAS-. This was accomplished by using different classifiers, such as Support Vector Machine (SVM), Grading Boosting Machine (GBM), Neural Networks (NNET), and Random Forest (RF). Texture analysis can provide a quantitative assessment of tumour heterogeneity by analysing both the distribution and relationship between the pixels in the image. The objective of this research is to demonstrate that CT-based Radiomics can predict the presence of mutation in the KRAS gene in colorectal cancer. This is a retrospective study, with 47 patients from the University Hospital, with a confirmatory pathological analysis of KRAS mutation. The highest accuracy and kappa achieved were 83% and 64.7%, respectively, with a sensitivity of 88.9% and a specificity of 75.0%, achieved by the NNET classifier using the texture feature vectors combining wavelet transform and Haralick coefficients. The fact of being able to identify the genetic expression of a tumour without having to perform either a biopsy or a genetic test is a great advantage, because it prevents invasive procedures that involve complications and may present biases in the sample. As well, it leads towards a more personalized and effective treatment. | en |
dc.rights | Atribución 4.0 Internacional | |
dc.rights.uri | http://creativecommons.org/licenses/by/4.0/ | |
dc.title | CT Radiomics in Colorectal Cancer: Detection of KRAS Mutation Using Texture Analysis and Machine Learning | en |
dc.type | Journal Article | es |
dc.authorsophos | Gonzalez-Castro, V.;Cernadas, E.;Huelga, E.;Fernandez-Delgado, M.;Porto, J.;Antunez, J. R.;Souto-Bayarri, M. | |
dc.identifier.doi | 10.3390/app10186214 | |
dc.identifier.sophos | 39726 | |
dc.issue.number | 18 | es |
dc.journal.title | APPLIED SCIENCES (BASEL) | es |
dc.organization | Servizo Galego de Saúde::Estrutura de Xestión Integrada (EOXI)::EOXI de Santiago de Compostela - Complexo Hospitalario Universitario de Santiago de Compostela::Anatomía Patolóxica | es |
dc.organization | Servizo Galego de Saúde::Estrutura de Xestión Integrada (EOXI)::EOXI de Santiago de Compostela - Complexo Hospitalario Universitario de Santiago de Compostela::Radiodiagnóstico | es |
dc.page.initial | 6214 | es |
dc.rights.accessRights | openAccess | |
dc.subject.keyword | CHUS | es |
dc.typefides | Artículo Original | es |
dc.typesophos | Artículo Original | es |
dc.volume.number | 10 | es |
Ficheros en el ítem
Este ítem aparece en la(s) siguiente(s) colección(ones)
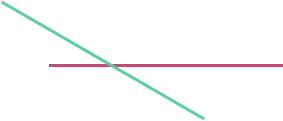