Identification of predictive factors of the degree of adherence to the Mediterranean diet through machine-learning techniques
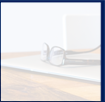
Identificadores
Identificadores
URI: http://hdl.handle.net/20.500.11940/16444
PMID: 33816938
DOI: 10.7717/peerj-cs.287
ISSN: 2376-5992
Visualización o descarga de ficheros
Visualización o descarga de ficheros
Fecha de publicación
2020Título de revista
PeerJ Computer science
Tipo de contenido
Journal Article
DeCS
máquinas de vectores de apoyo | trastornos nutricionales | estado nutricionalMeSH
Nutritional Status | Support Vector Machines | Nutrition DisordersResumen
Food consumption patterns have undergone changes that in recent years have resulted in serious health problems. Studies based on the evaluation of the nutritional status have determined that the adoption of a food pattern-based primarily on a Mediterranean diet (MD) has a preventive role, as well as the ability to mitigate the negative effects of certain pathologies. A group of more than 500 adults aged over 40 years from our cohort in Northwestern Spain was surveyed. Under our experimental design, 10 experiments were run with four different machine-learning algorithms and the predictive factors most relevant to the adherence of a MD were identified. A feature selection approach was explored and under a null hypothesis test, it was concluded that only 16 measures were of relevance, suggesting the strength of this observational study. Our findings indicate that the following factors have the highest predictive value in terms of the degree of adherence to the MD: basal metabolic rate, mini nutritional assessment questionnaire total score, weight, height, bone density, waist-hip ratio, smoking habits, age, EDI-OD, circumference of the arm, activity metabolism, subscapular skinfold, subscapular circumference in cm, circumference of the waist, circumference of the calf and brachial area.
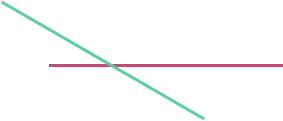